12. Experiments
12.3. Persistent Validity Problems: What You Still Need to Avoid
Learning Objectives
- Discuss the similarities and differences between the major social interaction threats to internal validity.
- Describe how a double-blind study design corrects for experimenter effects.
- Explain why an experiment should include a placebo in situations where participants have a belief in the treatment’s efficacy.
- Understand what external validity is and why it can be a problem for experiments.
To recap, a true experimental design includes both an experimental group that receives the stimulus and a control group that does not. As a result, the host of alternative factors that might potentially explain any change in our dependent variable—history, testing, instrumentation, maturation, regression—shouldn’t matter. Those factors should affect the control and experimental groups in a similar fashion, and therefore we can rule out causal stories other than our hypothesis that our stimulus brought about the observed outcomes. In turn, randomly assigning participants to the experimental and control groups minimizes the possibility that the two groups will be very different on any characteristic at the outset of the experiment, thus limiting the possibility of a selection effect. Incorporating these key checks within our experiment’s design improves its internal validity and allows us to draw firmer conclusions about whether a causal relationship between the independent and dependent variables actually exists.
That said, a classical experimental design remains at risk of various social interaction threats to internal validity. We’ll go through some of them below, and then talk about the larger vulnerability of experiments as a research method: its general lack of external validity. Please note that it is reasonable to expect any experimental study to have some problems of the sort described here, even if the experiment is rigorously designed. These limitations of internal and external validity should be acknowledged and discussed at length in the limitations section of your paper, but they do not necessarily condemn your study to irrelevance, and to some extent, these tradeoffs are inevitable in the pursuit of any research strategy, including an experimental design.
Social Interaction Threats
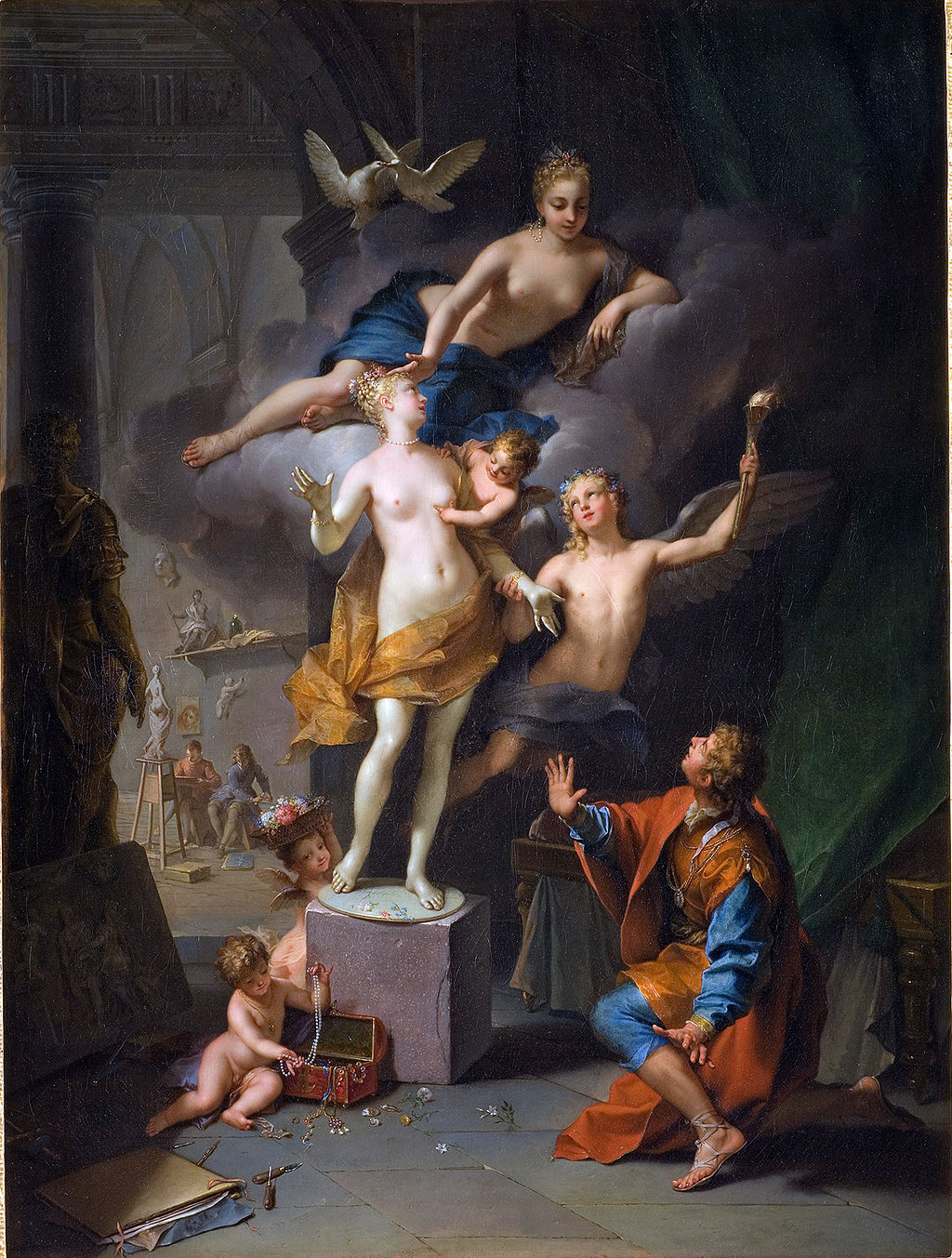
Social research is a social activity. People—researchers and participants—interact with one another at every stage in the process. This opens a window for sources of potential bias to creep into our experiments. Social interaction threats are potential problems with internal validity that arise as researchers and study participants interact with each other over the course of an experiment (Trochim 2020). In these situations, social pressures may generate outcomes in the lab setting that are not actually caused by the treatment itself. Most of these threats occur because the various groups or key people involved in carrying out the research are aware of each other’s existence and the role they play in the research project.
Here are the major social interaction threats to internal validity:
- Treatment diffusion and treatment imitation. Both of these threats can occur when members of a control group learn about the treatment being provided within the study and adapt their behaviors in response to that knowledge. In our math app example, let’s say the fourth-graders in the study’s experimental and control groups came from the same school. The students using the app might share their experiences with it at lunch or on the playground. One possibility is that the children in the control group—blown away by AddUpDog’s disruptive technology and canine-friendly interface—then decide to download the app on their own and use it avidly at home. As a result, there has been treatment diffusion across the two groups, even though the control group is not supposed to be exposed to the app. Another possibility is that the control-group kids get so excited about math apps in general that they stuff their phones and tablets with them and spend all their waking hours using them. In this case, members of the control group are engaging in treatment imitation—changing their math-learning behaviors based on their knowledge of the app-based tutoring the experimental group is receiving. Both of these examples of diffusion and imitation may affect the posttest performance of the control group, thereby jeopardizing our ability to calculate the direct impact that the math app has on students’ test scores.
- Compensatory rivalry and resentful demoralization. When compensatory rivalry is occurring, the control group knows what treatment the experimental group is getting and develops a competitive attitude with them. In our example, the fourth-graders in the control group might decide to compete with the experimental group “just to show them” how well they can do. In social contexts like these, participants may even be encouraged by well-meaning teachers or administrators to compete with each other. While this might make educational sense as a motivation for the students in both groups to work harder, it works against the researcher’s ability to determine whether the math app by itself has an effect on student performance. Resentful demoralization is basically the opposite of compensatory rivalry: here, members of the control group know what treatment the experimental group is receiving, but that knowledge doesn’t encourage them to compete—instead, it discourages them. They get frustrated and angry and give up. Unlike the previous threats we’ve just discussed, resentful demoralization is likely to exaggerate rather than mute posttest differences between groups. In other words, if the fourth-graders in our control group got fed up with adding up numbers after learning they weren’t selected to partake in the AddUpDog math experience, their poor scores on their posttest would make the app look even more effective than it actually is.
- Compensatory equalization of treatment. This social interaction threat directly involves a study’s researchers as well as participants. When the control and experimental groups become aware of the conditions that the other group is experiencing, they may yearn to be in the other group. Often they will put pressure on the experimenters to have them reassigned to the other group. Alternatively, the researchers may feel obligated to compensate one group for the advantages that the other group is seen to be receiving. Either course of action can muddy the experiment’s ability to accurately and precisely determine the impact of the treatment on the dependent variable if it winds up sabotaging the randomization process, artificially making the experimental and control groups more similar to each other, or otherwise undermining the study’s design.
- Experimenter expectancy. Researchers may also bias their study’s results if they expect the experimental and control groups to behave differently and then act in ways that further those expectations or make them known to participants. Let’s say we are fervent believers in the AddUpDog app and therefore are convinced that the math scores of the fourth-graders in our experimental group will shoot up after they use the app. If so, we researchers may take actions—consciously or unconsciously—that influence their behaviors and possibly the study’s findings as well. For example, we might unconsciously give the treatment group clearer instructions, more encouragement, or more time on the math exam they take for their posttest. That said, researchers don’t necessarily need to take specific actions that help the treatment group in order to create this form of bias—we may simply give off cues to our participants that make them try harder or slack off in line with our expectations. For instance, if the fourth-graders in our treatment group learn that they are supposed to perform better than the control group, they may feel inspired to do so. That same information might demoralize the control-group kids, affecting their actual performance on test day.
Note that this particular threat to internal validity arises first from the perceptions of the researchers (thus its name: experimenter expectancy effect). Somehow, these views then filter down to the participants themselves, who conform to researcher expectations. While you as an ethical researcher may swear up and down that you won’t put your thumb on the scale, studies have shown just how much a difference an experimenter’s expectations can make. Indeed, the experimenter expectancy effect is just one manifestation of a larger phenomenon called the Pygmalion effect (also known as the Rosenthal effect). The psychologists Robert Rosenthal and Lenore Jacobson (1966) coined the term after observing how teacher expectations strongly influenced student performance in the classroom. (In Greek myth, Pygmalion falls so intensely in love with a sculpture of a woman he carves that she comes to life.) In their own experiment at an elementary school, Rosenthal and Jacobson took students in 18 classes over six grades and randomly assigned a fifth in each class to the experimental group, with the remainder serving as the control group. For each of the students in the treatment group, researchers told their teachers that they would show “unusual intellectual gains” over the year. Lo and behold, even though these students had been assigned at random to the treatment group, they ended up showing greater gains in measured IQ than the control group did, especially in the lower grades. In a similar fashion, researcher expectations that experiment participants will behave in a certain fashion can easily become self-fulfilling.
Video 12.1. Experimenter Expectancy Effects. This video reviews some examples of how expectations of researchers can bias their studies in favor of confirmatory findings.
The social interaction threats we have described above can be minimized. One strategy is to recruit our experimental and control groups in a way that does not make them aware of the other group’s existence. For instance, we might pick our experimental group from one school, and our control group from another school (of course, this leaves our study open to the risks of selection bias we’ve discussed earlier). To avoid compensatory effects, we could make sure that everyone involved with the study knows how much the effectiveness of the experiment rests on preserving the original experimental and control groupings and not making any efforts to equalize outcomes across those groups. To deal with experimenter expectancy effects, we could conduct a double-blind study—not letting research staff who interact with participants know who is in the experimental or control group, and not letting the participants themselves know whether they are in one group or another. Both experimenters and participants are thus “blinded” to perceptions that might influence their actions and change the outcomes of the experiment. (A similar approach is utilized in academic peer reviews: scholars are typically asked to review manuscripts without being told the names of the authors, and the authors themselves are never told who their reviewers are, with the idea that this anonymity will prevent biases from influencing the conclusions of the reviewers.)
Table 12.1 summarizes all the threats to internal validity we have described in this chapter, along with possible solutions to them.
Table 12.1. Threats to Internal Validity and Ways to Address Them
Potential Bias |
Issue |
Solution |
History effect |
An occurrence outside the experimental setting influences the dependent variable, which could be mistaken for the direct effect of the stimulus or treatment. |
Add a control group; both the experimental and control groups should experience any extraneous events, allowing researchers to see the impact of the independent variable alone on outcomes. |
Maturation effect |
Changes we observe in the dependent variable between tests may have been caused by natural changes in our participants, rather than by the experimental treatment per se.
|
Add a control group; both the experimental and control groups should change or mature over time similarly, allowing us to separate the treatment effect from the effect of the passage of time.
|
Testing effect |
Giving our test subjects the pretest could influence their posttest scores. |
Add a control group; if both groups take the same pretest, the overall results won’t be affected. Using filler questions to hide the real purpose of the study can also help counter testing effects. |
Selection bias |
The experimental and control groups are different at the beginning of the study in ways that affect the study’s results. |
Randomly assign individuals to the control and experimental groups, which will make the two groups comparable. |
Regression to the mean |
Participants who score extremely high or extremely low on the pretest will tend to score closer to the middle (i.e., to the average or mean) on the next test, which may be mistaken for the impact of the treatment. |
Randomly assign individuals to the control and experimental groups. Both groups should now be equally subject to regression. |
Social Interaction Threats |
|
|
Treatment diffusion/imitation |
Members of the experimental and control groups may share their experiences, influencing outcomes for the control group. Control groups may imitate experimental treatments. |
Prevent contact between the experimental and control groups as much as possible. One way to accomplish this is by recruiting our experimental and control groups in a way that does not make them aware of the other group’s existence. |
Compensatory rivalry |
The control group knows what treatment the experimental group is getting and develops a competitive attitude with them. |
Prevent contact between the experimental and control groups as much as possible. One way to accomplish this is by recruiting our experimental and control groups in a way that does not make them aware of the other group’s existence. |
Resentful demoralization |
Members of the control group know what treatment the experimental group is receiving, and they become discouraged. |
Prevent contact between the experimental and control groups as much as possible. One way to accomplish this is by recruiting our experimental and control groups in a way that does not make them aware of the other group’s existence. |
Compensatory equalization of treatment |
Members of the control group learn about the conditions experienced by the experimental group and may put pressure on the experimenters to have them reassigned to the experimental group. Alternatively, the researchers may feel obligated to compensate one group for the advantages that the other group is seen to be receiving. |
Prevent contact between the experimental and control groups as much as possible. One way to accomplish this is by recruiting our experimental and control groups in a way that does not make them aware of the other group’s existence. |
Experimenter expectancy effect (Pygmalion effect) |
Researchers expect the experimental and control groups to behave differently and then act in ways that fulfill those expectations or make them known to participants. |
Set up a double-blind study in which neither the experimenters nor the participants know if who is in the experimental or control group. |
Trick or Treatment: How Best to Manipulate the Independent Variable
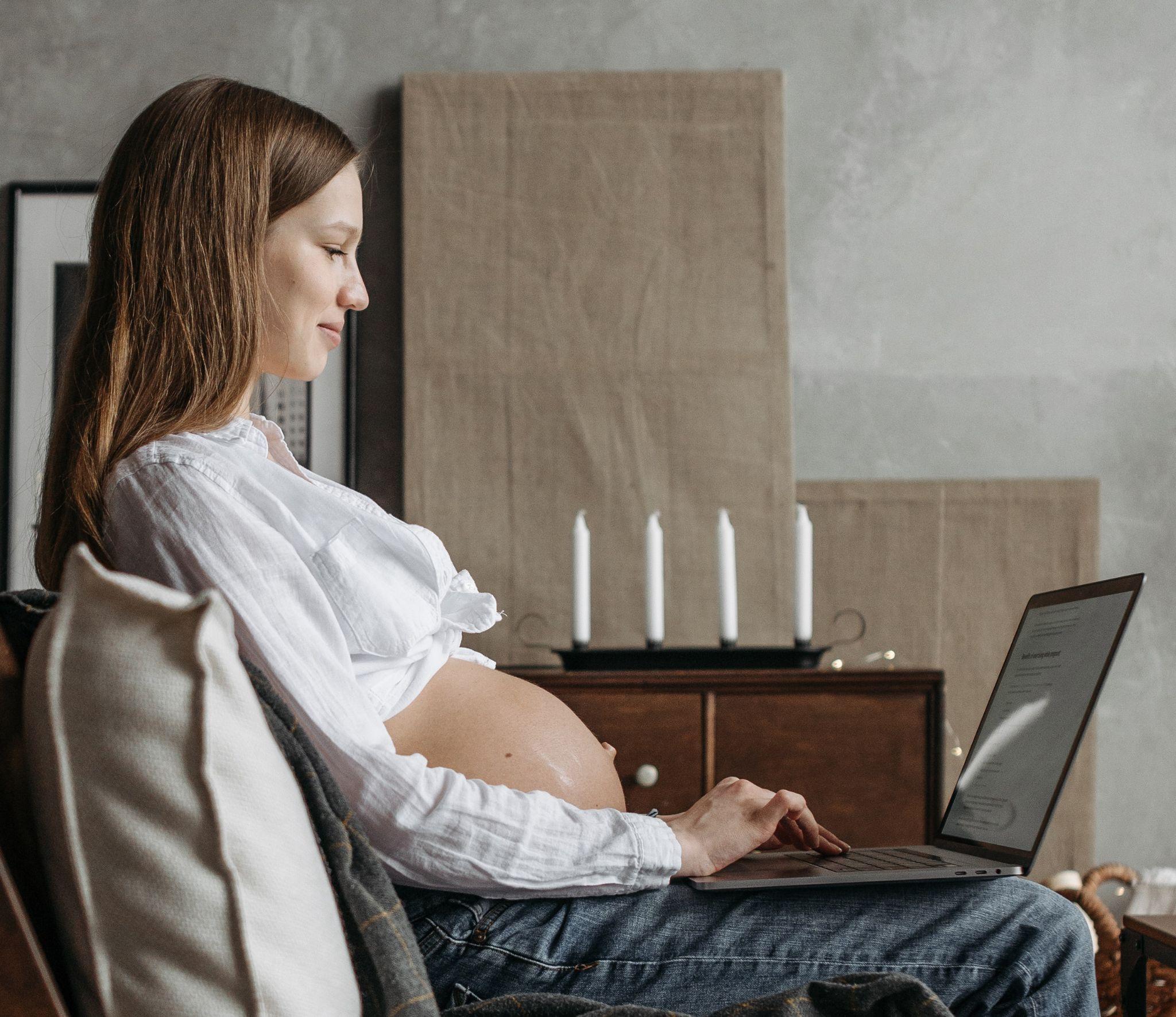
In a groundbreaking social psychological experiment, Shelley Correll, Stephen Benard, and In Paik (2007) sought to understand what was truly driving the “motherhood penalty”—the observed trend of women’s professional careers languishing after they have children. Social scientists had long debated two possible causes for this pause in career advancement and wage growth after women become mothers: (1) discrimination—that employers prefer nonmothers over mothers, believing that the latter won’t be as productive or committed to the job—or (2) lifestyle choices—that mothers opt to prioritize family over career, leading them reduce their efforts in the workplace and avoid pursuing more demanding (and therefore higher-paying) jobs.
Correll and her collaborators found a creative way to distinguish between these two possibilities and measure the impact of employer discrimination alone. In their lab, undergraduate participants were told that a California-based communications company was hiring a marketing director and wanted their feedback—as young and savvy media consumers—about whom to hire. They were individually presented with résumés and job application materials for two highly qualified women with comparable skills and experience. They were then asked to evaluate each candidate—scoring them on competence and commitment, deciding whether to recommend them for hire, and even choosing a salary offer within a set range.
Unbeknownst to the participants, the company was fictitious, and the (also fake) résumés were carefully constructed to be equally qualified, yet not suspiciously so. The “mother” résumé subtly flagged the fact that the candidate was a mother with a reference to their volunteer role as a parent-teacher association coordinator. In the “nonmother” résumé, that volunteering role was replaced by one not related to parenting—fundraising for a neighborhood association. Supplementary job application materials indicated that the “mother” candidate was married and had two children, while the “nonmother” candidate was only described as married. The researchers not only made the two fictitious job candidates appear equally qualified, but they swapped who was the “mother” and who was the “nonmother” for half of their participants—thereby using the magic of random assignment to further ensure the treatment (mother) and control (nonmother) scenarios were equivalent.
In spite of the commensurate qualifications of the two candidates, their evaluations were startlingly at odds. Mothers received competence ratings that were substantially lower than those for nonmothers. They were also recommended lower salaries. The researchers used the same approach with fictitious résumés of men and found that fathers actually did better than nonfathers on some measures, such as their perceived level of commitment. Together, these findings provided powerful empirical support for the claim that mothers are actually discriminated against in the job market. After all, the fake résumés were designed to present equivalent candidates who were randomly assigned as “parent” or “nonparent” to the participants who reviewed them. Any differences in perceived competence and commitment across the candidates could thus be attributed to the fact that the participants knew whether each candidate was a parent and—consciously or not—had their ratings influenced by that knowledge.
The elegant research design of the “motherhood penalty” study exemplifies a number of best practices for experiments. For one thing, the tight control that laboratory researchers have over the experimental setting was exploited fully in this study. Rather than just having participants talk vaguely about the professional competence and commitment of parents and nonparents, the researchers used an elaborate cover story and put together meticulously constructed fake job materials in order to come as close as possible to the decision-making process that might occur in an actual hiring manager’s office. (That said, we’ll have more to say later about how the contrived nature of lab experiments in general is a key weakness of this method.) The researchers also included a range of different measures of worker competence and commitment—from getting participants to explicitly rate those qualities on numerical scales, to asking them to provide hiring recommendations and salary offers, to even having them decide how many days of lateness would be tolerated before candidates were no longer recommended for hire (mothers were granted less time than nonmothers on that measure, too).
Another aspect of the study’s design that deserves praise is the sophisticated way the researchers manipulated their independent variable. The two templates of job materials were not only matched—that is, written to make the two candidates appear equally qualified—but each participant had an equal chance of receiving materials where Template A was the parent (and Template B the nonparent) or Template A was the nonparent (and Template B the parent). This combination of matching the parent and nonparent candidates and randomly mixing up their job materials meant that the only real difference between the two candidates was parental status. As a result, the researchers could confidently rule out any explanations for the motherhood penalty other than people’s stereotypes about working moms. Furthermore, by carefully and discreetly signaling parental status, the study ensured that what experimenters expected to find—that parenthood would matter in these hiring decisions—wasn’t overly obvious to participants and didn’t unduly influence their ratings.
Note, too, how the researchers essentially used a placebo in their experiment. As you probably know, randomized controlled trials to test the efficacy of drugs or other medical treatments will not simply give the experimental group the treatment and the control group nothing. A clinical drug trial will instead have the control group receive a sugar pill—ineffective and harmless—so that neither group knows whether they are receiving the actual drug. As doctors have long known, believing you are receiving an effective treatment will improve your health outcomes regardless of whether the treatment really works (again, expectations matter!). Using a sugar pill or its relevant equivalent avoids this placebo effect, a benefit not due to the treatment but rather the recipient’s belief in that treatment. By offering this “trick” or “treatment” pill at random, clinical researchers can make sure they are isolating the effect of the intervention and not capturing any other factors entangled with it.
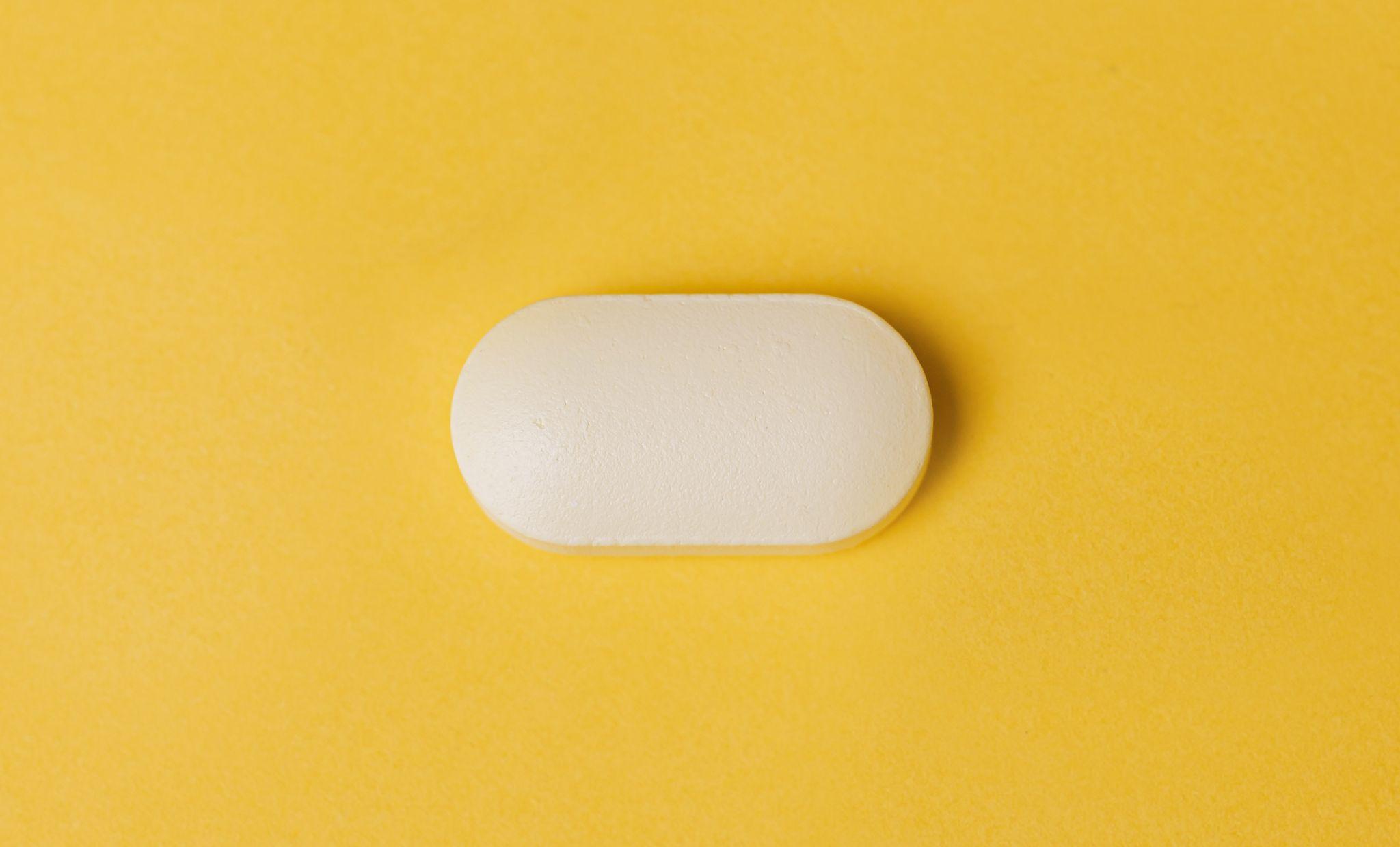
For similar reasons, social science researchers need to make sure their experimental and control groups have the exact same experience within the lab except for the presence or absence of the stimulus being studied. In the “motherhood penalty” study, the researchers didn’t have the “parent” résumé say that the job candidate was a PTA coordinator while leaving that space blank on the “nonparent” résumé. They created another volunteer role for the nonparent—fundraiser for a neighborhood association. Had they not added the fundraiser role, a skeptic might wonder if the lower scores that mothers received was due to their additional volunteering responsibility—which the nonmothers did not have, and which employers might see as conflicting with their work obligation. In other words, not being meticulously precise in their operationalization of the treatment would have meant the researchers were not really testing the effect of parental status, but rather parental status and something else.
These details might seem minor, but they are the sorts of considerations that good researchers fret about when designing their experiments. Setting up the ideal lab environment to study a social phenomenon and effectively manipulating the independent variable within that setting require a great deal of precision and creativity. And even when you think you have gotten those things right, whether your lab results hold up in the real world is a more intractable problem, as we will discuss next.
Problems with External Validity: When Your Results Do Not Generalize
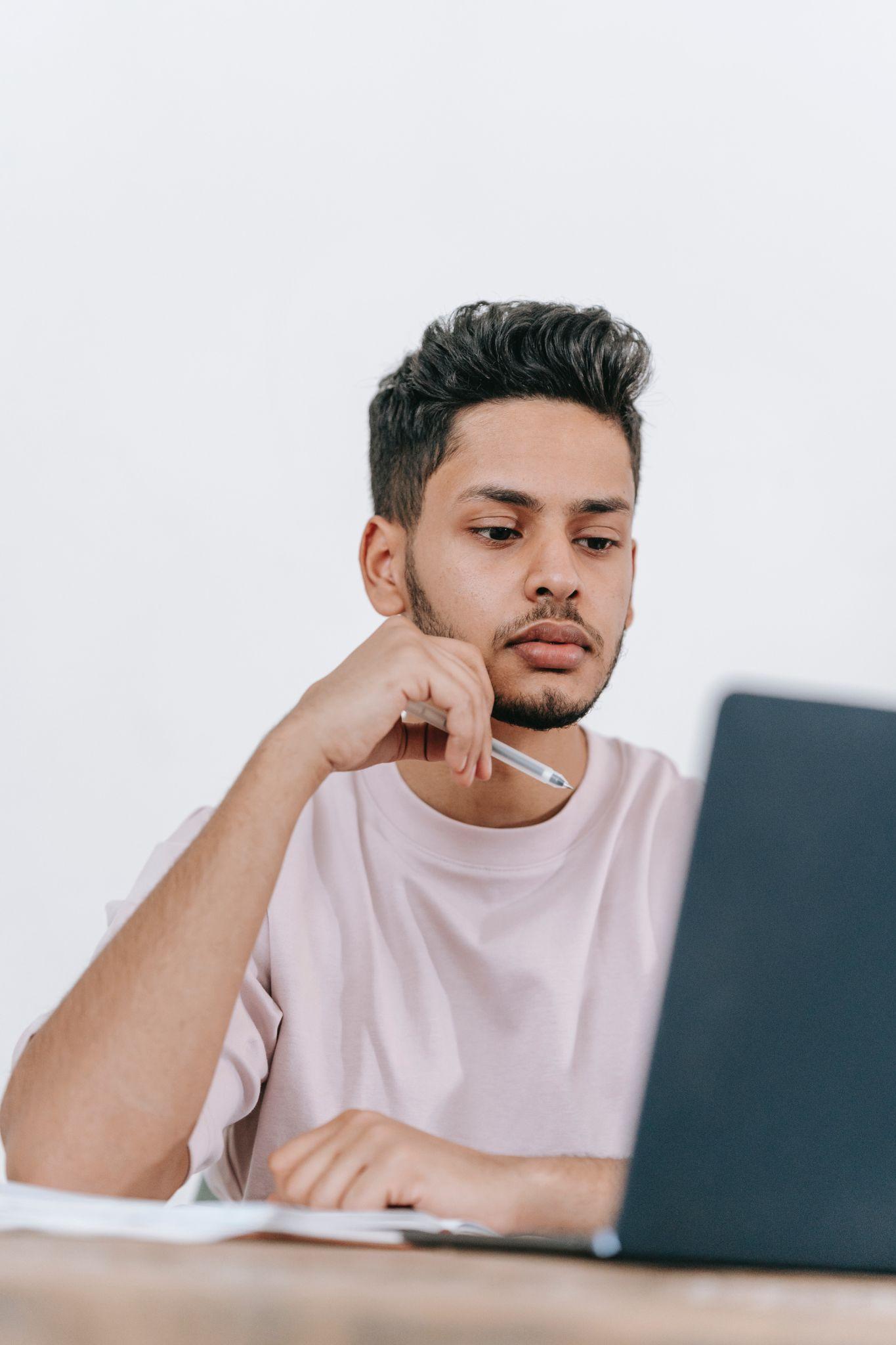
As we noted in the introduction to this chapter, external validity is the Achilles’ heel of the experimental method—a major vulnerability in an otherwise remarkably rigorous approach to social science. To expand on our previous definitions, external validity (also known as generalizability) refers to whether we can reasonably say that the results we observed in our study’s sample would also hold up in the target population. More broadly, we might wonder if we could generalize our results to another population entirely (say, Canada if our sample came from the United States), or another social context (a Muslim-majority culture if we studied a Christian-majority culture), or another time period (today if our data is from twenty years ago).
Laboratory experiments are criticized for their contrived conditions. In many psychology experiments, the participants come to a classroom or lab to fill out a series of questionnaires or perform a carefully designed computerized task, perhaps after experiencing a mock social interaction or watching a video of one. Yes, the researchers may try their best to emulate a real-world social situation, but a lab experiment is by definition artificial and only an approximation of actual life—and possibly a pretty poor one at that. The participant’s observations or actions in the lab setting may not reflect what would go on in the real world with real people interacting and real reputations or resources on the line. For example, Barbara Fredrickson and her colleagues (1998) conducted an experiment to study whether self-objectification produced body shaming, promoted unrestrained eating, and diminished math performance. They recruited undergraduate students to come to their lab on campus. Participants tried on a swimsuit or sweater alone in front of a full-length mirror. They then completed questionnaires on their attitudes toward body shaming and took a food taste test and a math test.
Does wearing a swimsuit before a mirror in a lab capture what it is like to feel shame about your body in real life? Would you actually experience some sort of body-shaming incident right before eating a meal or taking a test? Maybe, but the social scenario fabricated in this experiment is extreme in many ways, as it is in most lab studies. Their contrived situations can give us vital clues about how people think and act in their everyday lives, but they should also give us pause. For one thing, researchers need to be very clear about what can actually be generalized to the target population based on their experiment. They need to be aware of what we have previously described as a study’s scope conditions, the situations where a study’s findings can reasonably be thought to apply. For instance, our AddUpDog evaluation may have shown a causal effect, but under what conditions does that effect hold? We studied 10 hours of app usage over a week, and then tested math skills. Would fewer hours also have an effect? How long does any effect last? Would a different kind of math test give us different results? Would the app also work if we tested it on fourth-graders in another state or country? We don’t know the answers to these questions, given the narrow context and treatment involved in our single study—and lab experiments can be particularly narrow in the contexts and treatments they test. In any case, we as researchers need to be upfront about these potential limits to a study’s external validity.
It is also important to mention that the ability of experimenters to manipulate their independent variable in a valid and generalizable fashion often depends on subterfuge. In the “motherhood penalty” study, for example, participants were told they were providing feedback to a real startup, even though that was just a cover story. They were deceived in this way so that they would take their task of vetting the job candidates more seriously, better mimicking conditions in the real world. Some experiments will go even further, manipulating the conditions that participants experience by using confederates, individuals hired by the researchers (or sometimes the researchers themselves) who act in certain ways to maintain whatever cover story the study is using to test its treatment or stimulus. The use of confederates and deception more generally not only raises ethical issues of the sort we discussed in Chapter 8: Ethics, but it also runs the risk that participants see through the subterfuge, potentially undermining the study itself. In fact, a couple participants in the “motherhood penalty” study gave responses to researchers that raised concerns they might be suspicious of the cover story being used, prompting the researchers to remove them from the study to avoid social interaction threats of the kinds we discussed earlier.
Another issue of external validity that bedevils lab experiments is their tendency to draw upon a homogeneous population for their participants: specifically, college students from rich Western countries with major research universities. (We talked in Chapter 6: Sampling about how problematic these WEIRD samples can be.) College professors often have a subject pool available of students who must participate in a certain number of studies to meet a course requirement. Students can also be easily recruited on campus by posting descriptions of research studies—using in-person or online appeals—and offering a small amount of compensation. But the heavy reliance on this select group of experimental guinea pigs raises questions about whether the results of many lab experiments are broadly generalizable. By contrast, survey researchers usually recruit respondents directly from their populations of interest. They can easily defend the external validity of their research based on the mathematical principles of probability sampling.
To recap, laboratory experiments often run into problems with external validity because of (1) the contrived nature of the treatment and social context that researchers are trying to approximate within the lab setting; and (2) the use of homogeneous samples that may give us a false or skewed picture of social life in the real world. To deal with one or both of these concerns, some researchers choose to pursue field experiments as a sort of middle ground—an experiment conducted in the real world, often with larger and more representative samples. We’ll describe these types of experiments in the next section.
Deeper Dive: Problems with Pretests
We mentioned before that sometimes experimenters opt to drop the pretest and just conduct the posttest. After all, so long as they randomly assign their participants to the control and experimental groups and have sufficient numbers in both, they can reasonably expect the two groups to be fairly comparable. One benefit of this approach is that it saves resources that would have been spent pretesting both groups. Another is that the researcher avoids another potential problem of external validity, the interaction of testing and the experimental treatment (yes, it’s a mouthful).
If we use an experimental design with both a pretest and a posttest, we run the risk that any treatment effect we observe may not be just because of the influence of our independent variable, but rather because of the combination of the stimulus and the pretest given to the treatment group. Confused? Let’s make things more concrete with an example. Consider a social psychological experiment by Shannon K. McCoy and Brenda Major (2003) that sought to understand how perceptions of prejudice influence feelings of depression. All the study’s participants were given a pretest to assess their levels of depression, the researchers’ dependent variable. Participants then read an article suggesting that prejudice against a particular racial group was severe and pervasive. For participants randomly assigned to the experimental group, the racial group in question was their own racial group; for those in the control group, it was a group other than their own. As it turned out, the experimental group—those who read about pervasive prejudice against their own racial group—reported greater levels of depression than those in the control group.
What’s the problem here? We might be worried that giving participants a pretest to gauge their level of depression and then having them read about prejudice against their own ethnic group might tip them off to the relationship between prejudice and depression being studied. That, in turn, may make the information about racism that the participant just ingested more salient in their mind, leading them to score higher on the depression posttest. As a result, the experiment would not be testing just the impact of learning about prejudice against one’s racial group on one’s measured levels of depression. It would be testing the impact of learning about that prejudice and getting a depression test on one’s measured levels of depression.
This means we have an external validity issue: we cannot generalize, based on the results from this study, that reading about racism leads to greater depression, even though that’s really the causal relationship we wanted to assess. In the real world, people aren’t getting depression tests before they happen to read about racism. Therefore, the hit to their mental health that the treatment group suffered may not occur for them. The distinction may seem nitpicky, but it is implicit in the design of this study. To avoid this issue—the interaction of testing and the experimental treatment—we should think carefully about whether pretesting our participants will give them information that will affect their performance on the subsequent posttest. If so, the external validity of our study may be harmed. In that case, we may want to dispense with pretests (as illustrated in Figure 12.6) and instead rely on the power of randomization to ensure our two groups are similar at the outset in terms of any characteristic that might matter.
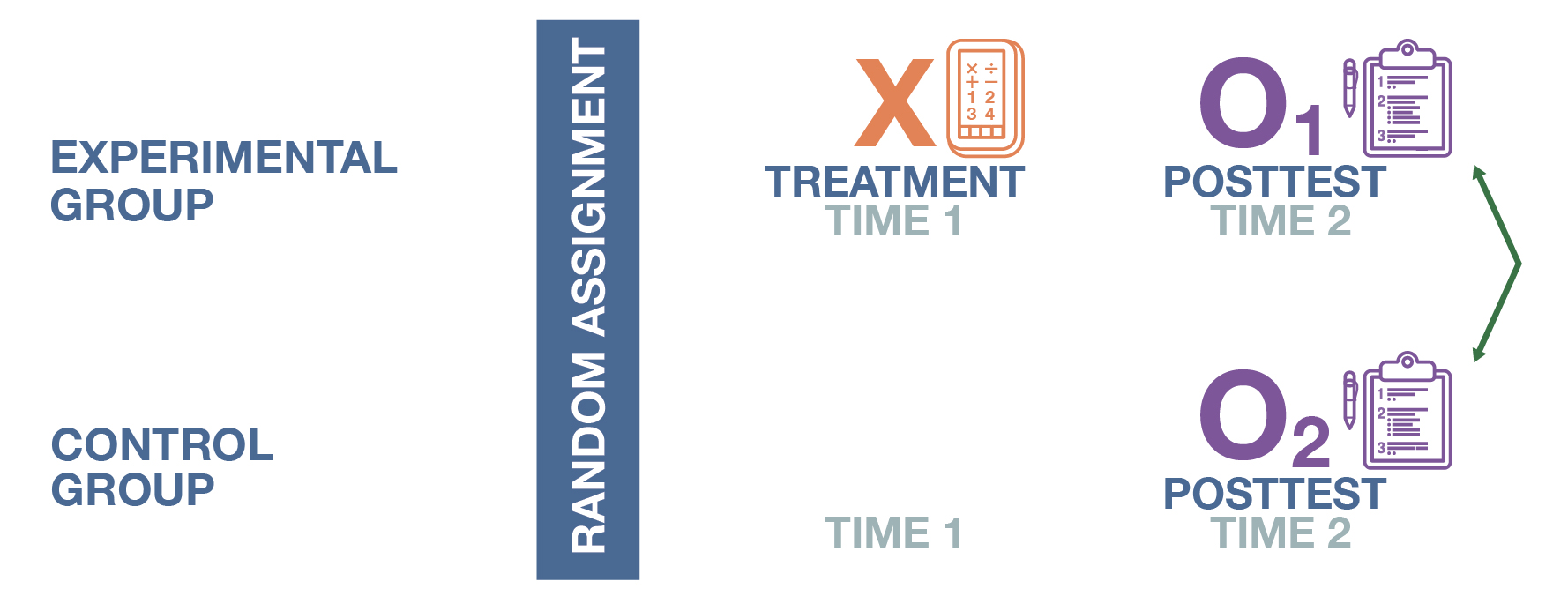
Key Takeaways
- Social interaction threats to internal validity include treatment diffusion, treatment imitation, compensatory rivalry, resentful demoralization, compensatory equalization of treatment, and experimenter expectancy effects.
- A double-blind study design corrects for experimenter effects because neither the experimenter nor the participant knows whether the participant is in the experimental or control group.
- If an intervention is given to an experimental group, the mere act of administering something to that group may trigger a response (a placebo effect). To rule out this effect, social scientific studies will have the control group undergo some sort of intervention other than the one being tested on the experimental group.
- Lab experiments cause issues with external validity because of (1) the contrived nature of the treatment and social context that researchers are trying to approximate within the lab setting; and (2) the use of homogeneous samples that may give us a false or skewed picture of social life in the real world.